Powering the future of
custom-built proteins.
Powering the future of purpose-built proteins.
Fable's innovative Machine Learning strategy harmonizes biology with computation, revolutionizing therapeutic development.
Our platform leverages extensive public and proprietary datasets housing billions of sequences, alongside protein structures, protein-protein interactions, all tied together through a sophisticated machine learning infrastructure. The result?
Precision-engineered biologics tailored for symmetry, shape, binding, affinity, potency, and developability.
The benefits of
purposeful design.
Our approach is designed to overcome current therapeutic index limitations and channel response where and when it matters.
This capability empowers us to craft innovative medicines tailored to tackle complex biological challenges.
Alanine Parallax
Precision platform
designed to solve
for complexity.
At the core, we utilize two proprietary foundation models: a structure-based model and a sequence-based model.
Through precision engineering at the atomic scale, our platform learns patterns within the 3-D structures and amino acid sequences of proteins and protein complexes.
Structure
Our structure model is pre-trained on a large set of data, built on state-of-the-art architectures and optimal reasoning in 3D space.
Sequence
Our sequence model is pre-trained on billions of sequences, learning the languages of biologics.
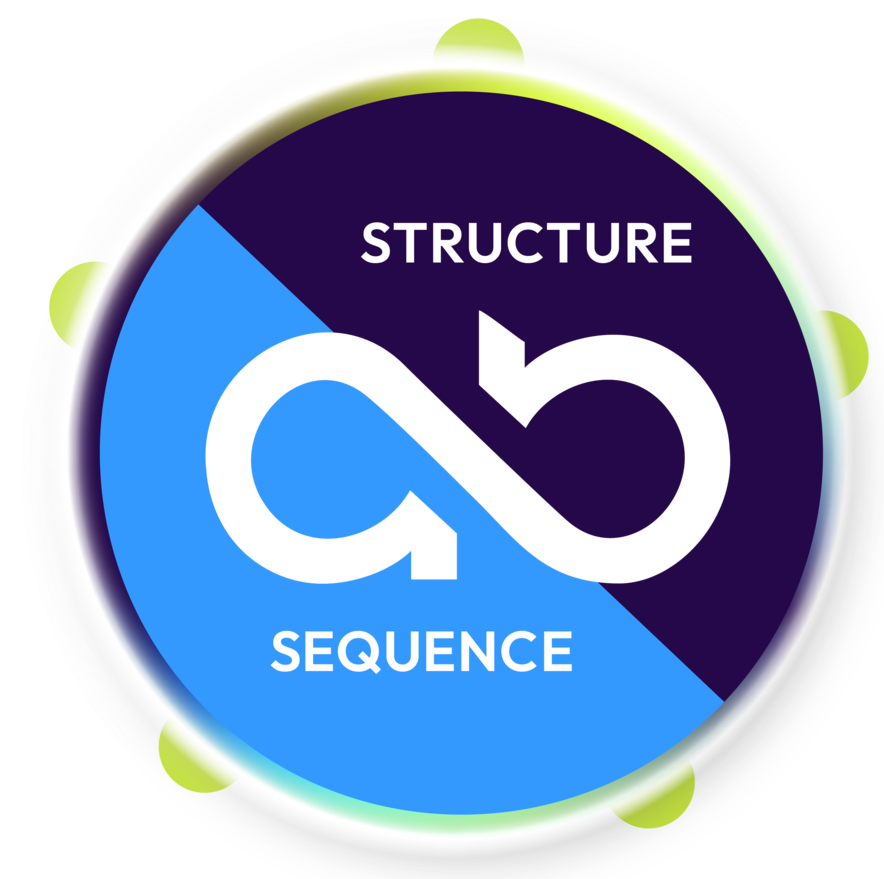
De novo design or optimization - what would you like your protein to do? We can do either.
De novo design
- Diffusion sub-model
- Sequence sub-model
- Scoring sub-model
optimization
- Optimization sub-model
- Scoring sub-model
“The pace of progress
in the field is astounding.
At Fable, we remain at the
forefront and are
well-positioned
to take optimal advantage of
these rapid developments.”

Setting our
platform apart.
Our 7 key differentiators drive precise protein design, seamlessly integrating binding and developability criteria into lead candidates.
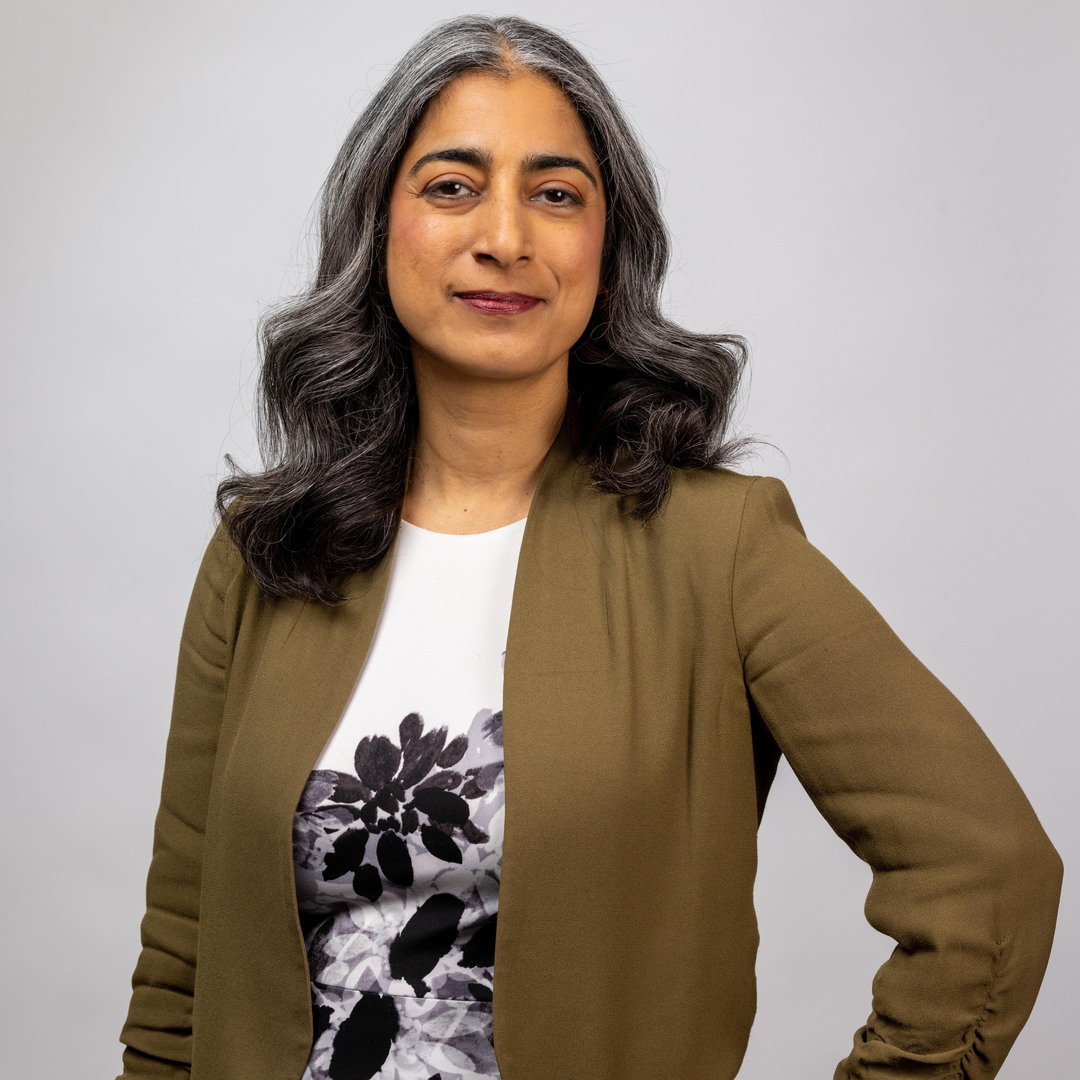
“Powering an ML platform
to deliver better medicines
requires an unrelenting
focus at the bench to
continuously generate data
of the highest quality.”
We've assembled a team with diverse and specialized skill sets, uniquely equipping us to explore the fast-paced world of protein design and drive forward new possibilities in medicine.
Arginine Parallax




